Machine Learning (ML) is an important enabler for optimizing, securing and managing mobile networks. This leads to increased collection and processing of data from network functions, which in turn may increase threats to sensitive end-user information. Consequently, mechanisms to reduce threats to end-user privacy are needed to take full advantage of ML. We seamlessly integrate Federated Learning (FL) into the 3GPP 5G Network Data Analytics (NWDA) architecture, and add a Multi-Party Computation (MPC) protocol for protecting the confidentiality of local updates. We evaluate the protocol and find that it has much lower overhead than previous work, without affecting ML performance.
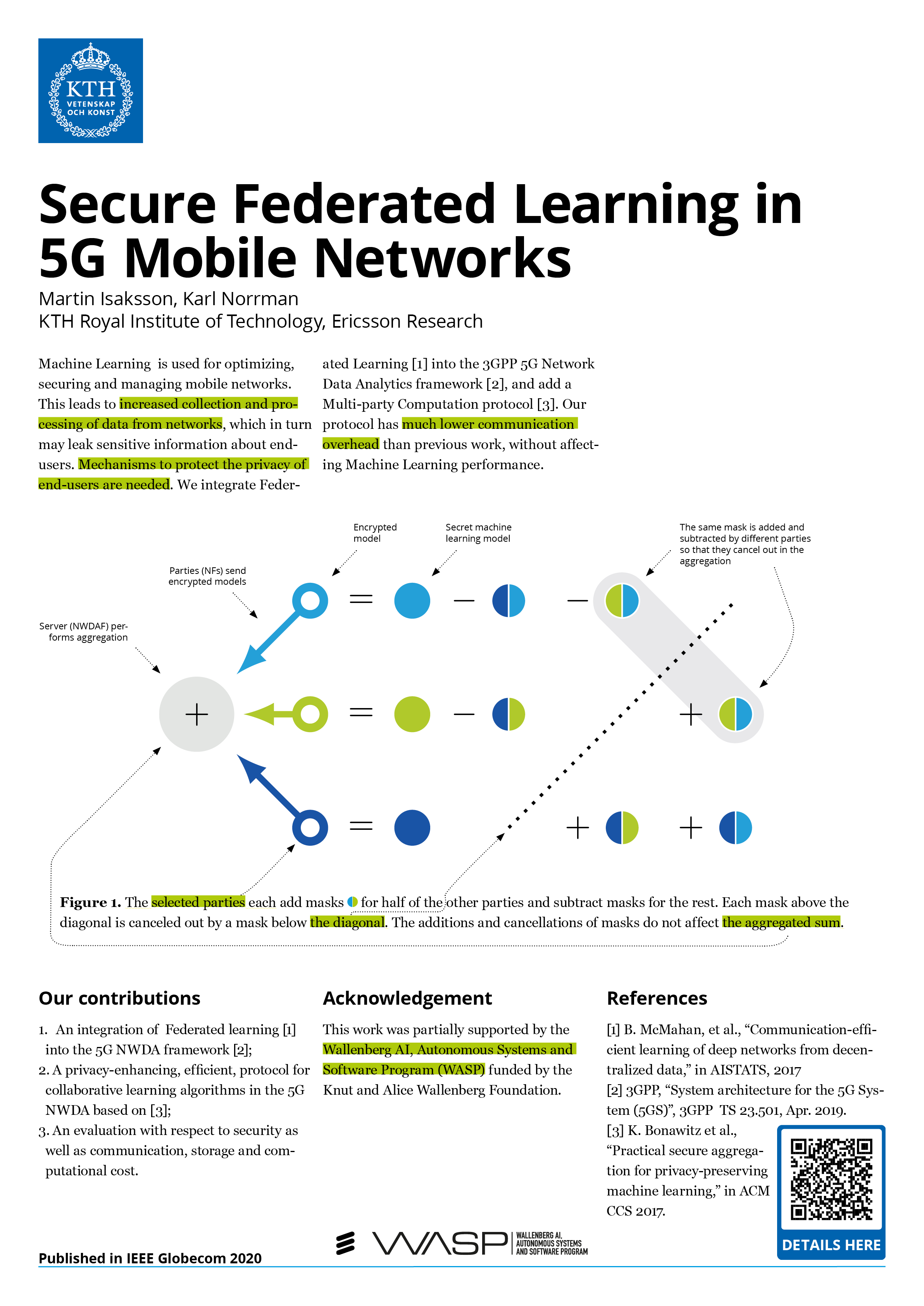
Secure Federated Learning in 5G Mobile Networks poster.
Background
This is a video for a technical report done for the 2020 WASP Project Course.
Suggested Citation
@INPROCEEDINGS{9322479,
author = {Martin Isaksson and
Karl Norrman},
title = {{Secure Federated Learning in 5G Mobile Networks}},
booktitle = {GLOBECOM 2020 - 2020 IEEE Global Communications Conference},
year = {2020},
url = {https://ieeexplore.ieee.org/document/9322479},
month = {December},
pages = {1-6},
doi = {10.1109/GLOBECOM42002.2020.9322479}}
}